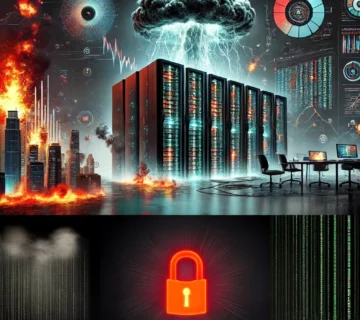
8 Major IT Disasters of 2024: Lessons for Business Continuity
Eight Major IT disasters of 2024, examining their causes and impacts across various sectors. Examples include widespread software failures affecting millions of computers, major outages at telecommunication companies and retailers, AI chatbot malfunctions, and government system errors. The article highlights the significant financial and reputational consequences of these incidents. Key takeaways emphasize the importance of rigorous software testing, robust system architecture, dependable third-party vendors, and ethical AI development to prevent future disruptions. Finally, it promotes a company's services for mitigating such risks.
... Read More